Recently, my favorite INSEAD Professor, Kevin Kaiser, came over to LinkedIn with a group of executives from around the world. We chatted about value creation in Silicon Valley. Like no other part of the world, Silicon Valley has seen stunning success, with a positive reinforcing cycle of startup success and funding opportunities (see the Startup Genome blog here for more information). Why? Because Silicon Valley creates value that changes our world. Let’s look at professional social networks. The following is solemnly the opinion of Kevin Kaiser and myself and not necessarily the opinion of my employer LinkedIn, one of the biggest professional social networks.
Professional Social Networks = Economic Enablers
Let’s look at the comparison as such. Is a professional network connecting business opportunities to people comparable at all to a bank or a financial market? Yes, because there is no distinction between human capital and financial capital as such. Any given object (for example, take your mobile phone, where you are reading this article) is only possible because of human capital. Those who followed the demise of the various anti-market experiments, most notably the Soviet Union or more recently Venezuela, witnessed what happens when a large percentage of human capital becomes misallocated.
Transparency Through Professional Social Networks
A professional network . In a perfect world, every one of us would find the perfect job in a domain where we excel and are most effective and create the most overall value our customers, ourselves, our company and thus, society as a whole. There is room for improvement – 70% of the US workers are disengaged (read more about this here).
Consider the potential of LinkedIn, as an example. Each of their 347 million members have documented their skills and careers online. This data enables LinkedIn to understand which position requires what kind of skills. “Who should one hire?” LinkedIn matches candidates to jobs, and predicts the best matches for each opportunity with their member profiles. Based on their data, LinkedIn can go even further and suggest potential career paths as far as 5 years down the road (Click here to read about how LinkedIn predicted the future of a Mashable reporter). By using data, LinkedIn offers transparency, and as anyone with a finance background knows, transparency improves the allocation process, resulting in less perceived risk with better returns.

Transactional Layer
There is another parallel between today’s professional networks and financial markets. They provide a transactional layer. Before the onset of banks, it was difficult to do business. The cost of a transaction was high and the process was complex. Banks helped to standardize the process, similarly to what we now see happening with professional networks. They make the process of searching and applying for a new job easy (Career-switcher? Read about the data driven approach – which industry should you choose?)
We are living in a world of creative destruction and constant change, resulting in an onslaught of new and innovative products and methods. This requires a workforce capable of continuous adaptability, performing tasks and jobs, which has never before existed. Who knows which skills will be valuable to society in the next 20 years? In a few hundred years, we have progressed from approximately 90% of our population working in farming, while food was scarce and the quality poor, to only 2%. This has enabled the other 98% to create entirely new industries, many of which resulted in the technological innovations, which made food more plentiful and affordable and accessible to more people than ever before, not to mention the advancements in areas from medical care to entertainment which enrich all of our lives in immeasurable ways. But as we “fire the farmers,” it is imperative that they find these new jobs and develop the skills to perform in them. There is a need for a “transactional layer”. Thus, by making it easier to search, find and apply to any job, we can react better to change as a society.
Data is the new LIFEBLOOD
The key to these improvements is data. This was recently well-described by Ann Winblad, co-founder and managing director of Hummer Winblad Venture Partners, in a recent interview: “I went from our alumni site on LinkedIn and to the LinkedIn created site and looked at the density of data they had on every one of the alum. Then I started digging and digging and digging in LinkedIn and realized, it’s not a human resource company, it’s a data company.” Data is what Jeff Weiner (@jeffweiner) describes as the Economic Graph. This dataset shows how members, companies, jobs, skills, schools and content are connected together.
The concern for PRIVACY
While the potential benefit of analyzing data to society is immense, it always brings up questions about privacy. These are valid concerns because as much as I want my bank data not to be public, I would like to keep the data conclusions of my professional network data private. It is not yet clear how to achieve such a level of privacy. Should we just stop economic opportunity by interdiction? An example I described in my book, “Ask Measure Learn” (Get your free sample chapter is here) is Schufa, the German credit-rating company, and the Hasso-Plattner-Institut from the University of Potsdam (HPI). Together, they started to investigate whether data from social networks could help to reduce credit risk. They planned on doing a test to analyze whether information on social networks such as Facebook and Xing (German LinkedIn) could help to predict credit worthiness.
Imagine the impact. If a financial provider can better assess your creditworthiness through data provided from social networks, the overall risk in providing loans would go down. Money would become cheaper and more accessible to more people. This is the core recipe for a booming economy. The Nobel Peace Prize-winning Garmeen Bank showed us this exact scenario. They did not use Facebook information, but they leveraged social networks formed by villages.
The project in Germany was stopped due to public outrage about privacy concerns. History however has taught us that the act of prohibition never really worked well. But there are companies like Wonga or Prosper both founded by the “who is who” in Silicon Valley who aim to disrupt the banking space with social network information.
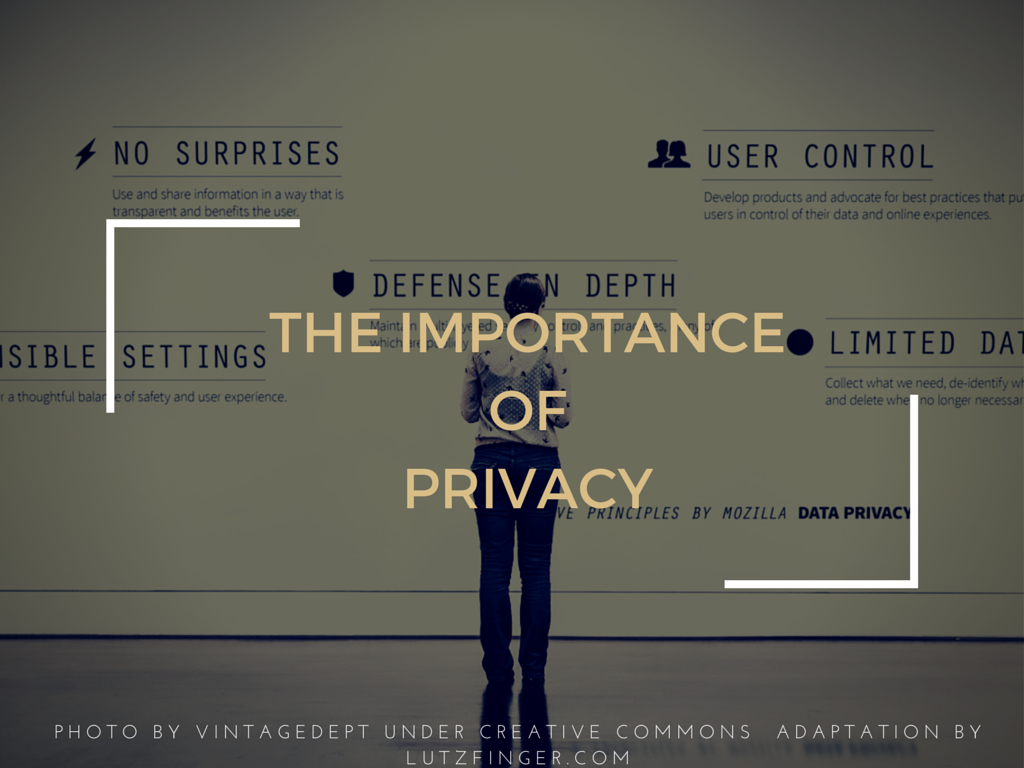
THE ROAD AHEAD: Data is the future
Professional networks are only the beginning. More areas of our life await the transparency that can be created with data. President Obama announced DJ Patil as the first Chief Data Science Officer of the White House at this year’s Strata. Their aim is to foster more business models that can foster opportunities and reduce risk in our economy with open data, even though the questions about privacy are not yet solved. The opportunities are amazing or as Jeff Weiner (CEO from LinkedIn) notably said it… “The creation of economic opportunity might be the defining issue of our time.”
This Column was co-authored by Lutz Finger, Director of Data Science at LinkedIn and author of the book “Ask Measure Learn” by O’Reilly Media and Kevin Kaiser, Professor of Management Practice, INSEAD, and co-author of “The Blue Line Imperative” and “Becoming a Top Manager”.
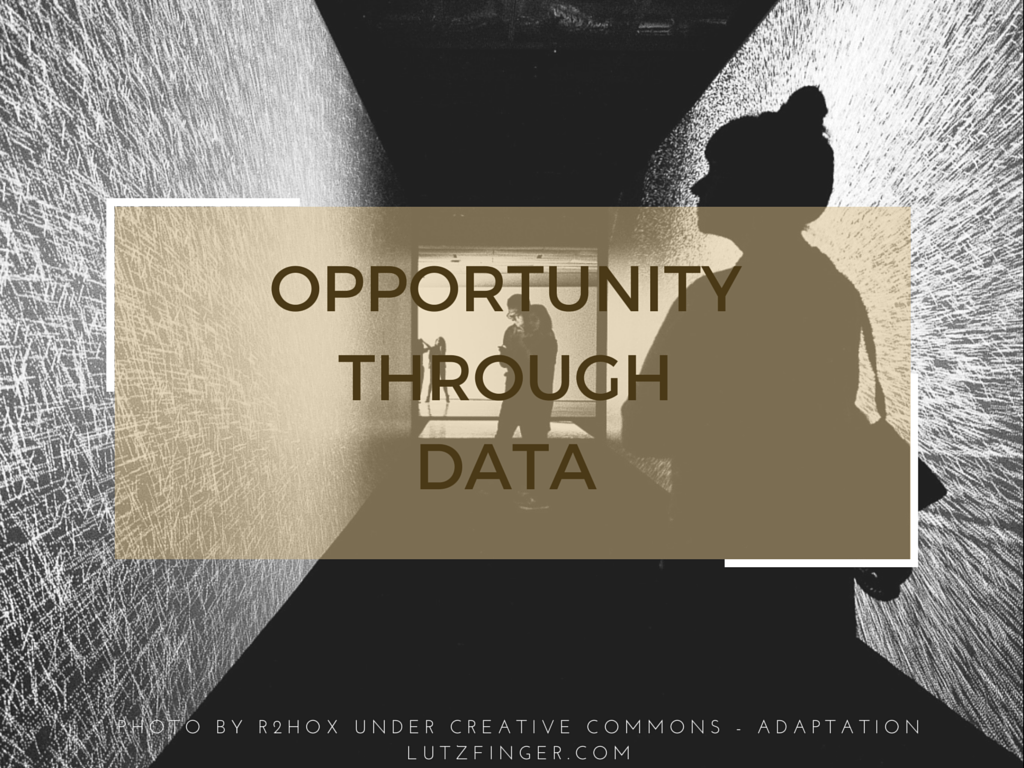
(republished from Forbes)